As was expected, there has been a change in direction that my corpus is headed. Originally, my plan was to analyze the lyrics of songs that are considered either happy or sad. My goal was basically to see what the factors are in these songs that make them considered to be either happy or sad. This would be interesting, but I decided to go a step further and add to my corpus of songs the top 40 songs in the US, United Kingdom, and Australia. Now, once I gather all of the trends that make a song happy or sad, I can apply this knowledge to the top 40s of the various countries and see what kind of trends are most commonly used in each country. Fortunately, Spotify has playlists with all of the top 40s for these countries. I decided to pick the countries that I did because they are all english speaking, which gets rid of the translation issue that I would have had with most other countries. I will try to keep all of Hofstede’s Cultural Dimensions in mind as I analyze my results to see how they are intertwined in the type of lyrics that are used.
Using both AntConc and Voyant to analyze these texts have led to some interesting findings. So far, on AntConc, I have only been using word frequency tools of words that I think would be significant. This, is not a great way for me to analyze the texts because I am using my bias to think of words to look for. Right now, the only significant finding I have using AntConc is that there are more female pronouns used in “happy” songs than “sad” songs. There is actually almost no female or male pronouns used in “sad” songs. This could be as a result of song writers realizing that people can be sad about a lot of things and decided to make them less people specific and more internalized.
I found Voyant’s collocation tool to be particularly attractive. This tool was able to show me words that are most frequently repeated. I found that words repeated in the “Sad” songs included “know”, “cry”, “love”, “used”, “mind”, and “I’m”. Most of these words can tell us a little bit about what the songs talk about. We see that “cry” is used, which is a pretty standard word that would be expected in a sad song. The word “used” is also frequently repeated. This could be telling us two things. Either that the songs are about someone or something feeling or being used, which could very well be an element of a sad song. It could also mean that the songs are talking about the way things “used” to be. This could be targeting the listener by trying to trigger old memories that may cause them to consider the song “sad”. Words repeated in the “happy” songs include “la”, “love”, “time”, “I’m”, “say”, and “like”. “La” being something that is repeated frequently can show us that lyrics could be less important in these “happy” songs and they are more about the melody and catchiness. What I noticed is that “love” is repeated frequently in both of these groups of songs.
“Because love makes you happy and love makes you sad” – Professor Katie Faull
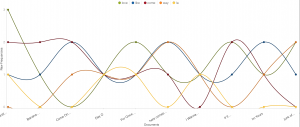
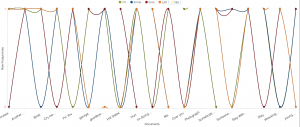